seven yevale's blog : Edge Computing for Data Science
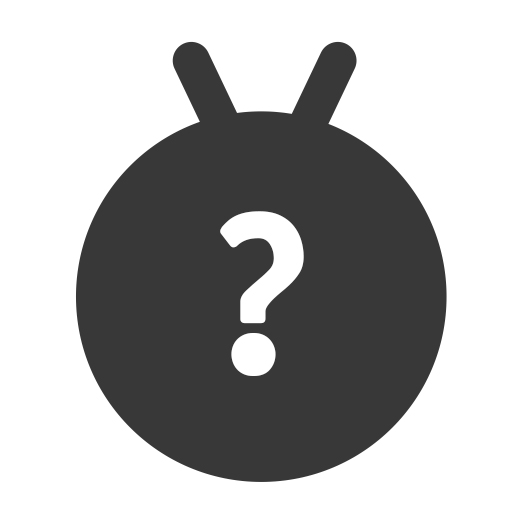
In the era of data-driven decision-making, organizations face the challenge of processing and analyzing vast amounts of data while ensuring real-time insights and low-latency responses. Edge computing has emerged as a transformative technology that brings computation and analytics closer to the data source, enabling faster and more efficient data processing. In this blog post, we will delve into the world of edge computing for data science, exploring its fundamentals, benefits, applications, and its potential to revolutionize the way we leverage data. Visit Data Science Course in Pune
Understanding Edge Computing:
Edge computing involves performing data processing, analytics, and decision-making closer to the data source or the "edge" of the network, rather than relying solely on centralized cloud servers. By decentralizing computation and bringing it closer to where the data is generated or consumed, edge computing reduces latency, bandwidth requirements, and improves overall system efficiency.
Benefits of Edge Computing for Data Science:
Real-Time Insights: Edge computing enables data scientists to derive real-time insights from streaming data sources, allowing for immediate responses and decision-making. By processing data at the edge, organizations can quickly detect patterns, anomalies, and trends, leading to more timely and accurate actions.
Reduced Latency: Edge computing significantly reduces the time it takes to transmit data to a centralized cloud server for processing. This low-latency environment is crucial for applications that require real-time analytics, such as autonomous vehicles, industrial automation, or IoT-enabled systems.
Bandwidth Optimization: By processing and filtering data at the edge, edge computing minimizes the amount of data that needs to be transmitted to the cloud for further analysis. This optimization of bandwidth usage reduces costs and alleviates network congestion, especially in scenarios with limited connectivity or high data volumes.
Improved Data Privacy and Security: Edge computing can enhance data privacy and security by minimizing the exposure of sensitive data. Instead of sending data to the cloud for processing, edge devices can perform local data analytics, ensuring that sensitive information remains within the organization's boundaries. Learn more Data Science Classes in Pune
Applications of Edge Computing for Data Science:
Internet of Things (IoT): Edge computing is particularly relevant in IoT deployments, where numerous devices generate vast amounts of data. By performing data processing and analytics at the edge, IoT devices can respond quickly to local events, trigger actions, and provide real-time insights without relying heavily on cloud connectivity.
Smart Cities and Infrastructure: Edge computing enables the development of smart city solutions by processing sensor data from various sources, such as traffic cameras, environmental sensors, and smart meters. By analyzing data at the edge, cities can optimize traffic management, improve energy efficiency, and enhance public safety.
Healthcare and Telemedicine: Edge computing has significant potential in healthcare applications, where real-time monitoring, analysis, and response are critical. By leveraging edge devices, healthcare professionals can collect and analyze patient data in real-time, enabling early detection of health issues, remote monitoring, and timely interventions.
Edge Analytics in Manufacturing: Edge computing revolutionizes manufacturing processes by enabling real-time monitoring and analysis of sensor data from production lines and equipment. This allows for predictive maintenance, quality control, and optimization of manufacturing operations, leading to increased efficiency and reduced downtime.
Challenges and Considerations:
Scalability: As the number of edge devices increases, managing and scaling edge computing infrastructure becomes more challenging. Organizations must design scalable architectures and implement effective device management strategies to handle the growing volume of edge devices and data.
Data Governance and Compliance: Data governance becomes crucial in edge computing environments, as data is distributed across multiple edge devices. Organizations must ensure compliance with data protection regulations, implement secure data transmission protocols, and establish clear policies for data access and storage.
Resource Constraints: Edge devices often have limited computing power.
Read more Data Science Training in Pune.
- Career
- News
- Technology